10.12.23-AI-Driven-Panel
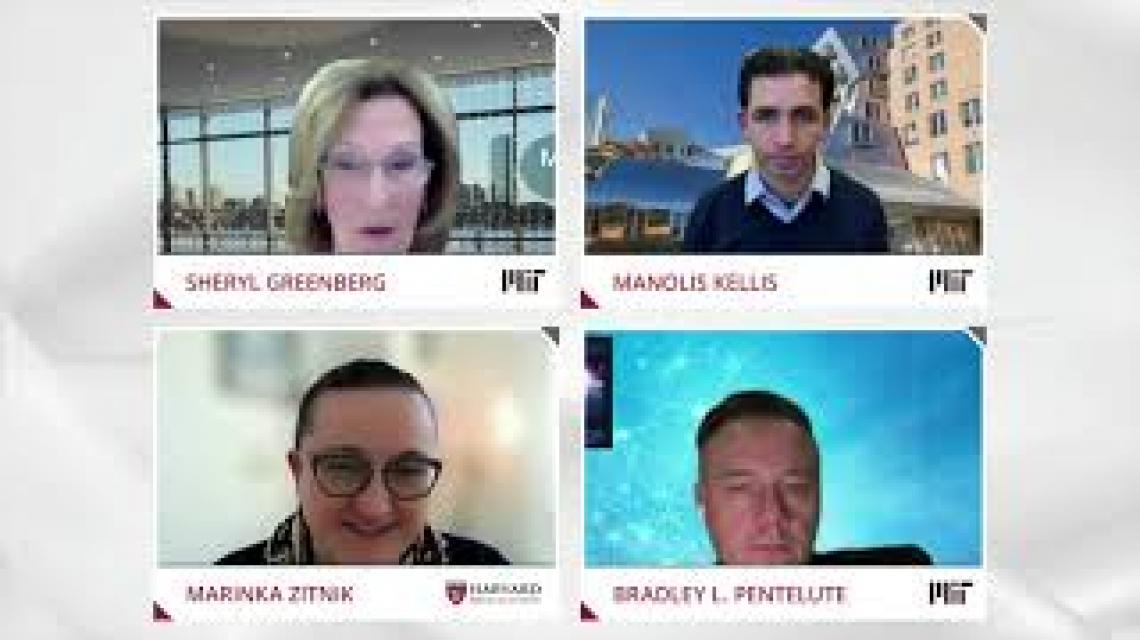
-
Video details
Panel Discussion
-
Interactive transcript
SHERYL GREENBERG: Now we're going to start our panel discussion. So I'd like to invite Brad and Marinka to come back and join us for a discussion on AI-driven drug discovery. Again, please feel free to put your questions in the Q&A. And if it's meant for Brad, Marinka, or Manolis, please put their name on the question.
Sorry. So I guess what I'd like to do is-- there's so much here. And it's really hard to figure out how it all fits together, although there's obvious places. Maybe you could each give us a two-minute perspective on how you see things fitting together. Manolis, we can start with you.
MANOLIS KELLIS: So there's many ways in which this all fits together. And there's many ways in which we would love to engage with both ILP members and members of the audience here who might not yet be members. So one way is to actually get engaged with the ILP program and become members and have more interactions.
The other way is to actually-- for the specific therapeutic area that you are the most interested in, basically, reach out. Collaborate. We can start individual collaborations with both established pharmaceutical companies, as well as new biotech startups, to, A, understand better the specific therapeutic areas that you're interested in-- basically, being able to use our extraordinary pipeline that you saw here across chemistry, AI, and genomics, genetics to dissect patient samples through our network of collaborators to understand how are these pathways altered to open up and expand that area of biology, and to also go after specific therapeutic modalities-- specific molecules, specific target genes-- to start dissecting these pathways and applying this pipeline.
As you saw from the examples across Brad, Marinka, and I, our methods are extremely general. They can apply across cancer, neurodegeneration, psychiatric disorders, immune disorders, metabolic disorders, cardiac disorders. And we really believe that this is a general platform that can be used to truly make a difference in human disease within our lifetimes. We really hope to not just understand, but truly eradicate a large number of these genes that we can already have a handle for using these methodologies. So that's what we're very excited about. That's where it all fits in together between genetics, genomics, chemistry, and AI. So who wants to go next?
SHERYL GREENBERG: Great. Marinka?
MARINKA ZITNIK: Yeah. So Manolis already very nicely pointed out opportunities across different types of biomedical data and also therapeutic modalities. In addition to that, from the AI perspective, there are numerous specific therapeutic tasks, from nominating drug targets in a potentially cell type-specific manner, to designing protein molecular structures and do that conditional on other biological targets, to be matching and extracting multi-omic molecular signatures from patients and then from patient-level data, and matching that to therapeutic benefits.
So, across the drug discovery and development pipeline, there are these critical sets of tasks or decisions that are required to be made. And those tasks can be formulated as valid machine learning tasks that require the development of state-of-the-art and novel models that have fundamentally better capabilities than what we are currently having.
So we're very excited about the opportunities to collaborate on the front of, really, designing new and better models; thinking about how those models are optimized towards biomedically and biochemically relevant endpoints, and what would those endpoints even be; and, finally, integrating that into experimental platforms that both Brad and Manolis' lab are developing and have, so that we have well-integrated systems that couple experiments with simulation and real world data across scales in AI models.
SHERYL GREENBERG: Thank you, Marinka. Brad?
BRADLEY L. PENTELUTE: Yeah, no. I couldn't agree more with everything that was already said. I just-- I always imagine this-- very simply, let's connect a computer, some AI, and genetics, along with chemistry, and, eventually, we'll end up really nominating molecules that impact human health. So that's the world we're in.
We'll ask-- really want to come up with a cure for Alzheimer's disease. All of a sudden, your prompt will go into an algorithm. It'll spit out all these potential steps. It'll feed into what Marinka's been pioneering. Well, now, here's everything you've got to consider. Here are some potential molecules. It's probably not going to be perfect. It's going to tell us there's 5,000 different possible molecules.
Then it'll come on to a robot in our lab that will make all these molecules, and it'll feed back into a cell assay or what have you that then will capture this whole new data set from Manolis's group and so forth. And then it'll feed back into the algorithm based on what we learned from that experiment. And, eventually, it'll be one molecule that we think will actually end up changing the world. So that's really what I think-- how this whole strategy will be brought together.
MANOLIS KELLIS: And I have to say that collaboration is key. Basically, we're not going to do this alone. That's where my last slide comes in, in terms of the coalition. And that's why we're so excited to be working with ILP and with all of you guys. Just seeing 500 responders for this webinar is just so exciting across so many different industries. And academia is not going to do it alone. Industry is not going to do it alone.
We really need to build bridges between the two and to bring the stakeholders together, to bring the hospitals, the patients, the doctors with engineering, with biology, with chemistry, with AI. And I think it's the biggest challenge of our generation-- to finally be able to tackle and wrestle down human disease. And there's so much suffering in the world that we can-- it is within our capabilities right now to start eradicating.
SHERYL GREENBERG: Terrific. So question for you all is-- how do you decide what diseases, what approaches need to be worked on first? Because there's a lot to be done, obviously, in every single one. And, as you pointed out, there are multiple drivers.
MANOLIS KELLIS: So if you look at the World Health Organization, you basically have what are the most important diseases. And what are they? Number one-- cardiac disease. Number two-- Alzheimer's. Number three-- basically, obesity and type 2 diabetes and all the related disorders. So, of course, we're going after those. And cancer is somewhere in there, like number 1, 2, depending on where you look. And that's the other one that we're going after.
So we are not shy to take on things that were previously thought of as unpackable. And I think that the field has matured enormously, and we're finally ready to take on those large challenges. But, at the same time, in parallel with working on those dramatically large and complex and multifaceted disorders, we're also working on rare diseases to basically couple these common and these rare facets that are all targeting the same underlying pathways.
And that's one of the things that we're so excited about-- to be able to work side by side with things that are extremely polygenic, extremely multifactorial-- to split them up into their hallmarks and their pathways through the functional genomics, the integration, the modular biology, and the systems-level views, and then, at the same time, to take these monogenic disorders that are extremely well specific and then to use those as a conduit for finding molecules that can modulate these pathways. And, of course, I'd love to hear Marinka's view, who's doing so much in this aspect of connecting the rare and the common. Marinka, go ahead.
MARINKA ZITNIK: Yeah. I think it's crucially important to take this cross-disease view that can help us-- actually also help with decision making in terms of where are the opportunities that could potentially maximize the success of the discovery and development. And so that is important because it can-- for certain diseases that have been studied extensively, yet with very little or limited therapeutic success, we can break down those complex diseases into more manageable molecular phenotypes, and then study those individual phenotypes, develop solutions for them, and then think about composing those solutions at the pathway level to find solutions for bigger diseases.
And, if we have this kind of a mechanism that can help us break down complex diseases and study them in the context of a large number of molecular phenotypes across different omics data, that can help us understand better both complex diseases-- rare diseases that can help describe some of complex diseases as a set or mixture of rare disease phenotypes across different biological scales, and can help more manageable solutions-- manageable way-- study these really complex combinatorial pathways, and then eventually build solutions in a composable, modular way, which ultimately can provide insights across many disease phenotypes.
And so a large part of our work on the AI front is to be able to go across a number of disease phenotypes and see-- what are the commonalities? What are the differences? How can those commonalities be explained across different levels of biological data, across different cell types, across different ways of molecular different types of molecular interactions? And then see what are, then, the opportunities that could help address specific disease phenotypes, and whether they can be easily reused across different diseases.
SHERYL GREENBERG: Thank you, Marinka.
MANOLIS KELLIS: I can add something here to basically say that-- it might sound extremely ambitious to say, listen, we're going to take on all these pathways across all of these different diseases. But this is simply the nature of the beast. This is simply how disease actually works. Alzheimer's doesn't give us the luxury of just going after one pathway. There are truly about a dozen pathways that are very clearly and reliably and reproducibly, over and over again, coming up when we're studying Alzheimer's disease.
Moreover, it's not just a luxury to study a single disease at a time. Because, when you study lipid metabolism, you find it in obesity. You find it in Alzheimer's. You find it in cardiac disorders. You find it in cancer. You basically have this extraordinary, number one, complexity of any one disease; number two, reuse of any one pathway.
And, frankly, the only way to truly understand the complexity of disease is to study, in a systems-level way, all of these different facets at the same time. Because, if you only study one of them, you'll be fooling yourself, thinking that that's the one driver, when, in fact, there's so many additional pathways. So I think that's where the systems-level, systematic approach is both possible and, in some ways, necessary to truly make an impact.
MARINKA ZITNIK: And if I can just add to what you just said-- for example, in a recent study with [INAUDIBLE] model where we were-- after providing AI-based diagnoses for patients that could not be diagnosed with traditional medicine and to standard approaches, we were asking, why could not those patients be diagnosed easily? And it is because, when you look at a group of patients that might eventually-- were diagnosed with the same disease-- they do not share any direct visible genetic components if you are simply looking at the overlap of the variants.
But, when we start looking at their variants-- at the level of molecular pathways and phenotypes that those variants are influencing-- all of a sudden we can start seeing similarities and commonalities between those patients. And we can put our patients [INAUDIBLE] type of capability of models to easily retrieve and find what our patients with similar phenotypic genetic features, and do that in a much more versatile, flexible manner by not only looking at direct overlap between variants, but also looking at how those variants propagate to molecular pathways, and then what are the molecular pathway, or knowledge graph, view. And what is knowledge graph overlap between those phenotypes is what was enabling us, and enabling the AI model to be able to provide predictions that were actually correct.
SHERYL GREENBERG: So a question, then-- what is the fastest way to demonstrate the power of your combined approaches and expertise and this collaboration? Does it make sense to start with the potential for already approved therapeutics because you don't have to wait for approval, or is there a better way?
MANOLIS KELLIS: It's funny that you say that because we're holding meetings constantly between the three of us. And, in a few days, we're actually having this jamboree to go after a small number of pathways and a small number of molecules. And some of them are going to be reusing existing molecules to basically demonstrate either a new target or a new modality of utilization.
In some cases, it's going to be combination treatments, where we basically find that one molecule is pushing the system one way, but then something is missing. And we are adding another molecule to either restore or add additional movement, if you wish, of pushing either towards or away from the effect of the first molecule. And then the third way is designing actually new therapeutics.
And that's where all of these AI modeling and all of this chemical synthesis comes in-- so I would say a little bit of a combination of those to basically tackle specific pathways that we currently have very strong leads for. And I tried to show some of them in my presentation, and I think Brad and Marinka also showed in theirs. So I think that's our way forward. Brad, would you like to add?
BRADLEY L. PENTELUTE: Yeah, no. I totally agree. I think that's totally right. There were a couple of questions that came up, too, that we only have five minutes that we probably want to get through them. I thought they were-- yeah. Sheryl, if you wanted to quarterback that?
SHERYL GREENBERG: Sure. Absolutely. So one question from the audience is, could you please comment on the status of machine learning-guided drug gene delivery? We all know that LNPs and messenger RNA may need many more years to become the hero of combating pandemics. However, LNP discovery is still a labor-intensive task now, especially for IV delivery. And what are your perspectives-- how best to use machine learning to guide delivery systems or drug products instead of drug substances?
BRADLEY L. PENTELUTE: Yeah. That's really like the venue that I walk through. And it's real. Machine learning is going to be able to guide drug products. There's no question. I think that, essentially, you'll have a certain specification with a certain number of modules. And you'll essentially use machine learning to tell you how to bring them all together. And, certainly, even in the case of programmable medicines, oligonucleotide-based therapies, genetic medicines, and so forth, that delivery technologies are being built.
I think the data sets are emerging. We're seeing it happen. I just walked through a really, really nice story. In doing that, we're also working with machine learning to essentially nominate ligands-- Marinka and Manolis and so forth-- about what tissues we want to target and what genes we want to knock down. So that's all going to end up turning out to be in a modular format.
SHERYL GREENBERG: And can you predict adverse effects of therapies using the same technologies?
BRADLEY L. PENTELUTE: I think that's on Marinka.
MARINKA ZITNIK: I'm happy to comment on that. So it's a great, incredibly important question. So we are developing AI models that can tackle these questions on two fronts-- first, on the molecular design phase, where we develop models that are multi-objective optimizers that can simultaneously optimize molecular structure for multiple criteria. And those criteria also include minimizing toxicity of the structures that the model is building and creating over a series of iterative ML refinement.
On the other end of the spectrum, we are leveraging large population health data sets, such as adverse event reporting system at the population level produced by FDA, our counterpart counterparts of FDA in Europe, as well as in Asia, with the goal of going with this large population-scale data sets to tease out adverse events that are often severe, rare, or only seen in patient populations once drugs are approved.
And that kind of data can be used as an effective prior on the models in order to predict adverse events of existing FDA-approved therapeutics. But then that knowledge can be transferred to novel drugs in development based on different kind of data that allow us to relate existing drugs to novel drugs in development. And so we're very much interested in pursuing this direction as well, in modeling adverse events and safety of individual drugs.
And then drugs in combination-- considered together jointly in combinations of two or even three drugs, where we can start asking questions about whether a drug that is already on the market can be effectively combined with another drug to either mitigate known safety issues or, in a combination, improve overall quality of life for patients.
MANOLIS KELLIS: You're muted, Sheryl.
SHERYL GREENBERG: Sorry. Thank you so much for that, Marinka. We have time for one more question. And, historically, biotechnology has required extended investments, despite higher uncertain returns. With the convergence of various technologies, it appears that the financial landscape may also evolve with the industry. What forms of financing do you believe are most essential in this collaborative effort to effectively combat diseases?
MANOLIS KELLIS: So I'm happy to take a first stab at this. And the idea is the following. Many companies, again, are focused on a single molecule. And they're basically putting all of their eggs in that one basket. I feel that the risk is, of course, higher, even though the reward could be enormous. But, in any one of them in isolation, the risk might be unsustainable for investors.
Andrew Lo at MIT in the Lab for Financial Engineering, who's a very close collaborator of our team, has basically turned this model on its head and said, listen, if I instead tell you that, if I take 20 shots on goal, that my success probability is going to be almost certain to eventually hit 1 as I start combining these independent opportunities for therapeutics, then, suddenly, it becomes a much more appealing investment.
And I think what's really unique about our team here is that, because we have such a platform-- technology-- to take on so many independent pathways using this multimodal approach of reusing both the capabilities of the AI and the genomics and the circuitry and the chemistry across all of these different therapeutic modalities and targets and pathways, that allows us to learn in a much more confident way about the probability of success and effectively hedge our bets-- mitigate the risk across multiple therapeutic programs simultaneously.
The second problem is that a lot of the knowledge has to be built independently in the current model of these single shot on goal for the existing therapeutic platforms that are basically, then, constantly having to rebuild the knowledge from scratch every time one of those drugs fails.
The ability of this collaboration here is to now share knowledge across these different disease areas in multiple different collaborations, where we can actually learn from these multiple shots on goal, and also share the knowledge to basically increase the probability of each of these shots succeeding. So these are the two dramatically different capabilities here, which I believe make this combined approach slightly different from the traditional approach.
SHERYL GREENBERG: That sounds like a good approach to me. And I think, with that, we'll wrap up and close. And I'd like to thank each of our presenters today for your excellent presentations and for the excellent discussion-- Brad, Marinka, and Manolis. I'd also like to thank our video and communications team for running this webinar, and you, our viewers, for your attention and your participation. Please feel free to reach out to us. Thank you, and be well and safe.
MANOLIS KELLIS: Thank you, Sheryl.
-
Video details
Panel Discussion
-
Interactive transcript
SHERYL GREENBERG: Now we're going to start our panel discussion. So I'd like to invite Brad and Marinka to come back and join us for a discussion on AI-driven drug discovery. Again, please feel free to put your questions in the Q&A. And if it's meant for Brad, Marinka, or Manolis, please put their name on the question.
Sorry. So I guess what I'd like to do is-- there's so much here. And it's really hard to figure out how it all fits together, although there's obvious places. Maybe you could each give us a two-minute perspective on how you see things fitting together. Manolis, we can start with you.
MANOLIS KELLIS: So there's many ways in which this all fits together. And there's many ways in which we would love to engage with both ILP members and members of the audience here who might not yet be members. So one way is to actually get engaged with the ILP program and become members and have more interactions.
The other way is to actually-- for the specific therapeutic area that you are the most interested in, basically, reach out. Collaborate. We can start individual collaborations with both established pharmaceutical companies, as well as new biotech startups, to, A, understand better the specific therapeutic areas that you're interested in-- basically, being able to use our extraordinary pipeline that you saw here across chemistry, AI, and genomics, genetics to dissect patient samples through our network of collaborators to understand how are these pathways altered to open up and expand that area of biology, and to also go after specific therapeutic modalities-- specific molecules, specific target genes-- to start dissecting these pathways and applying this pipeline.
As you saw from the examples across Brad, Marinka, and I, our methods are extremely general. They can apply across cancer, neurodegeneration, psychiatric disorders, immune disorders, metabolic disorders, cardiac disorders. And we really believe that this is a general platform that can be used to truly make a difference in human disease within our lifetimes. We really hope to not just understand, but truly eradicate a large number of these genes that we can already have a handle for using these methodologies. So that's what we're very excited about. That's where it all fits in together between genetics, genomics, chemistry, and AI. So who wants to go next?
SHERYL GREENBERG: Great. Marinka?
MARINKA ZITNIK: Yeah. So Manolis already very nicely pointed out opportunities across different types of biomedical data and also therapeutic modalities. In addition to that, from the AI perspective, there are numerous specific therapeutic tasks, from nominating drug targets in a potentially cell type-specific manner, to designing protein molecular structures and do that conditional on other biological targets, to be matching and extracting multi-omic molecular signatures from patients and then from patient-level data, and matching that to therapeutic benefits.
So, across the drug discovery and development pipeline, there are these critical sets of tasks or decisions that are required to be made. And those tasks can be formulated as valid machine learning tasks that require the development of state-of-the-art and novel models that have fundamentally better capabilities than what we are currently having.
So we're very excited about the opportunities to collaborate on the front of, really, designing new and better models; thinking about how those models are optimized towards biomedically and biochemically relevant endpoints, and what would those endpoints even be; and, finally, integrating that into experimental platforms that both Brad and Manolis' lab are developing and have, so that we have well-integrated systems that couple experiments with simulation and real world data across scales in AI models.
SHERYL GREENBERG: Thank you, Marinka. Brad?
BRADLEY L. PENTELUTE: Yeah, no. I couldn't agree more with everything that was already said. I just-- I always imagine this-- very simply, let's connect a computer, some AI, and genetics, along with chemistry, and, eventually, we'll end up really nominating molecules that impact human health. So that's the world we're in.
We'll ask-- really want to come up with a cure for Alzheimer's disease. All of a sudden, your prompt will go into an algorithm. It'll spit out all these potential steps. It'll feed into what Marinka's been pioneering. Well, now, here's everything you've got to consider. Here are some potential molecules. It's probably not going to be perfect. It's going to tell us there's 5,000 different possible molecules.
Then it'll come on to a robot in our lab that will make all these molecules, and it'll feed back into a cell assay or what have you that then will capture this whole new data set from Manolis's group and so forth. And then it'll feed back into the algorithm based on what we learned from that experiment. And, eventually, it'll be one molecule that we think will actually end up changing the world. So that's really what I think-- how this whole strategy will be brought together.
MANOLIS KELLIS: And I have to say that collaboration is key. Basically, we're not going to do this alone. That's where my last slide comes in, in terms of the coalition. And that's why we're so excited to be working with ILP and with all of you guys. Just seeing 500 responders for this webinar is just so exciting across so many different industries. And academia is not going to do it alone. Industry is not going to do it alone.
We really need to build bridges between the two and to bring the stakeholders together, to bring the hospitals, the patients, the doctors with engineering, with biology, with chemistry, with AI. And I think it's the biggest challenge of our generation-- to finally be able to tackle and wrestle down human disease. And there's so much suffering in the world that we can-- it is within our capabilities right now to start eradicating.
SHERYL GREENBERG: Terrific. So question for you all is-- how do you decide what diseases, what approaches need to be worked on first? Because there's a lot to be done, obviously, in every single one. And, as you pointed out, there are multiple drivers.
MANOLIS KELLIS: So if you look at the World Health Organization, you basically have what are the most important diseases. And what are they? Number one-- cardiac disease. Number two-- Alzheimer's. Number three-- basically, obesity and type 2 diabetes and all the related disorders. So, of course, we're going after those. And cancer is somewhere in there, like number 1, 2, depending on where you look. And that's the other one that we're going after.
So we are not shy to take on things that were previously thought of as unpackable. And I think that the field has matured enormously, and we're finally ready to take on those large challenges. But, at the same time, in parallel with working on those dramatically large and complex and multifaceted disorders, we're also working on rare diseases to basically couple these common and these rare facets that are all targeting the same underlying pathways.
And that's one of the things that we're so excited about-- to be able to work side by side with things that are extremely polygenic, extremely multifactorial-- to split them up into their hallmarks and their pathways through the functional genomics, the integration, the modular biology, and the systems-level views, and then, at the same time, to take these monogenic disorders that are extremely well specific and then to use those as a conduit for finding molecules that can modulate these pathways. And, of course, I'd love to hear Marinka's view, who's doing so much in this aspect of connecting the rare and the common. Marinka, go ahead.
MARINKA ZITNIK: Yeah. I think it's crucially important to take this cross-disease view that can help us-- actually also help with decision making in terms of where are the opportunities that could potentially maximize the success of the discovery and development. And so that is important because it can-- for certain diseases that have been studied extensively, yet with very little or limited therapeutic success, we can break down those complex diseases into more manageable molecular phenotypes, and then study those individual phenotypes, develop solutions for them, and then think about composing those solutions at the pathway level to find solutions for bigger diseases.
And, if we have this kind of a mechanism that can help us break down complex diseases and study them in the context of a large number of molecular phenotypes across different omics data, that can help us understand better both complex diseases-- rare diseases that can help describe some of complex diseases as a set or mixture of rare disease phenotypes across different biological scales, and can help more manageable solutions-- manageable way-- study these really complex combinatorial pathways, and then eventually build solutions in a composable, modular way, which ultimately can provide insights across many disease phenotypes.
And so a large part of our work on the AI front is to be able to go across a number of disease phenotypes and see-- what are the commonalities? What are the differences? How can those commonalities be explained across different levels of biological data, across different cell types, across different ways of molecular different types of molecular interactions? And then see what are, then, the opportunities that could help address specific disease phenotypes, and whether they can be easily reused across different diseases.
SHERYL GREENBERG: Thank you, Marinka.
MANOLIS KELLIS: I can add something here to basically say that-- it might sound extremely ambitious to say, listen, we're going to take on all these pathways across all of these different diseases. But this is simply the nature of the beast. This is simply how disease actually works. Alzheimer's doesn't give us the luxury of just going after one pathway. There are truly about a dozen pathways that are very clearly and reliably and reproducibly, over and over again, coming up when we're studying Alzheimer's disease.
Moreover, it's not just a luxury to study a single disease at a time. Because, when you study lipid metabolism, you find it in obesity. You find it in Alzheimer's. You find it in cardiac disorders. You find it in cancer. You basically have this extraordinary, number one, complexity of any one disease; number two, reuse of any one pathway.
And, frankly, the only way to truly understand the complexity of disease is to study, in a systems-level way, all of these different facets at the same time. Because, if you only study one of them, you'll be fooling yourself, thinking that that's the one driver, when, in fact, there's so many additional pathways. So I think that's where the systems-level, systematic approach is both possible and, in some ways, necessary to truly make an impact.
MARINKA ZITNIK: And if I can just add to what you just said-- for example, in a recent study with [INAUDIBLE] model where we were-- after providing AI-based diagnoses for patients that could not be diagnosed with traditional medicine and to standard approaches, we were asking, why could not those patients be diagnosed easily? And it is because, when you look at a group of patients that might eventually-- were diagnosed with the same disease-- they do not share any direct visible genetic components if you are simply looking at the overlap of the variants.
But, when we start looking at their variants-- at the level of molecular pathways and phenotypes that those variants are influencing-- all of a sudden we can start seeing similarities and commonalities between those patients. And we can put our patients [INAUDIBLE] type of capability of models to easily retrieve and find what our patients with similar phenotypic genetic features, and do that in a much more versatile, flexible manner by not only looking at direct overlap between variants, but also looking at how those variants propagate to molecular pathways, and then what are the molecular pathway, or knowledge graph, view. And what is knowledge graph overlap between those phenotypes is what was enabling us, and enabling the AI model to be able to provide predictions that were actually correct.
SHERYL GREENBERG: So a question, then-- what is the fastest way to demonstrate the power of your combined approaches and expertise and this collaboration? Does it make sense to start with the potential for already approved therapeutics because you don't have to wait for approval, or is there a better way?
MANOLIS KELLIS: It's funny that you say that because we're holding meetings constantly between the three of us. And, in a few days, we're actually having this jamboree to go after a small number of pathways and a small number of molecules. And some of them are going to be reusing existing molecules to basically demonstrate either a new target or a new modality of utilization.
In some cases, it's going to be combination treatments, where we basically find that one molecule is pushing the system one way, but then something is missing. And we are adding another molecule to either restore or add additional movement, if you wish, of pushing either towards or away from the effect of the first molecule. And then the third way is designing actually new therapeutics.
And that's where all of these AI modeling and all of this chemical synthesis comes in-- so I would say a little bit of a combination of those to basically tackle specific pathways that we currently have very strong leads for. And I tried to show some of them in my presentation, and I think Brad and Marinka also showed in theirs. So I think that's our way forward. Brad, would you like to add?
BRADLEY L. PENTELUTE: Yeah, no. I totally agree. I think that's totally right. There were a couple of questions that came up, too, that we only have five minutes that we probably want to get through them. I thought they were-- yeah. Sheryl, if you wanted to quarterback that?
SHERYL GREENBERG: Sure. Absolutely. So one question from the audience is, could you please comment on the status of machine learning-guided drug gene delivery? We all know that LNPs and messenger RNA may need many more years to become the hero of combating pandemics. However, LNP discovery is still a labor-intensive task now, especially for IV delivery. And what are your perspectives-- how best to use machine learning to guide delivery systems or drug products instead of drug substances?
BRADLEY L. PENTELUTE: Yeah. That's really like the venue that I walk through. And it's real. Machine learning is going to be able to guide drug products. There's no question. I think that, essentially, you'll have a certain specification with a certain number of modules. And you'll essentially use machine learning to tell you how to bring them all together. And, certainly, even in the case of programmable medicines, oligonucleotide-based therapies, genetic medicines, and so forth, that delivery technologies are being built.
I think the data sets are emerging. We're seeing it happen. I just walked through a really, really nice story. In doing that, we're also working with machine learning to essentially nominate ligands-- Marinka and Manolis and so forth-- about what tissues we want to target and what genes we want to knock down. So that's all going to end up turning out to be in a modular format.
SHERYL GREENBERG: And can you predict adverse effects of therapies using the same technologies?
BRADLEY L. PENTELUTE: I think that's on Marinka.
MARINKA ZITNIK: I'm happy to comment on that. So it's a great, incredibly important question. So we are developing AI models that can tackle these questions on two fronts-- first, on the molecular design phase, where we develop models that are multi-objective optimizers that can simultaneously optimize molecular structure for multiple criteria. And those criteria also include minimizing toxicity of the structures that the model is building and creating over a series of iterative ML refinement.
On the other end of the spectrum, we are leveraging large population health data sets, such as adverse event reporting system at the population level produced by FDA, our counterpart counterparts of FDA in Europe, as well as in Asia, with the goal of going with this large population-scale data sets to tease out adverse events that are often severe, rare, or only seen in patient populations once drugs are approved.
And that kind of data can be used as an effective prior on the models in order to predict adverse events of existing FDA-approved therapeutics. But then that knowledge can be transferred to novel drugs in development based on different kind of data that allow us to relate existing drugs to novel drugs in development. And so we're very much interested in pursuing this direction as well, in modeling adverse events and safety of individual drugs.
And then drugs in combination-- considered together jointly in combinations of two or even three drugs, where we can start asking questions about whether a drug that is already on the market can be effectively combined with another drug to either mitigate known safety issues or, in a combination, improve overall quality of life for patients.
MANOLIS KELLIS: You're muted, Sheryl.
SHERYL GREENBERG: Sorry. Thank you so much for that, Marinka. We have time for one more question. And, historically, biotechnology has required extended investments, despite higher uncertain returns. With the convergence of various technologies, it appears that the financial landscape may also evolve with the industry. What forms of financing do you believe are most essential in this collaborative effort to effectively combat diseases?
MANOLIS KELLIS: So I'm happy to take a first stab at this. And the idea is the following. Many companies, again, are focused on a single molecule. And they're basically putting all of their eggs in that one basket. I feel that the risk is, of course, higher, even though the reward could be enormous. But, in any one of them in isolation, the risk might be unsustainable for investors.
Andrew Lo at MIT in the Lab for Financial Engineering, who's a very close collaborator of our team, has basically turned this model on its head and said, listen, if I instead tell you that, if I take 20 shots on goal, that my success probability is going to be almost certain to eventually hit 1 as I start combining these independent opportunities for therapeutics, then, suddenly, it becomes a much more appealing investment.
And I think what's really unique about our team here is that, because we have such a platform-- technology-- to take on so many independent pathways using this multimodal approach of reusing both the capabilities of the AI and the genomics and the circuitry and the chemistry across all of these different therapeutic modalities and targets and pathways, that allows us to learn in a much more confident way about the probability of success and effectively hedge our bets-- mitigate the risk across multiple therapeutic programs simultaneously.
The second problem is that a lot of the knowledge has to be built independently in the current model of these single shot on goal for the existing therapeutic platforms that are basically, then, constantly having to rebuild the knowledge from scratch every time one of those drugs fails.
The ability of this collaboration here is to now share knowledge across these different disease areas in multiple different collaborations, where we can actually learn from these multiple shots on goal, and also share the knowledge to basically increase the probability of each of these shots succeeding. So these are the two dramatically different capabilities here, which I believe make this combined approach slightly different from the traditional approach.
SHERYL GREENBERG: That sounds like a good approach to me. And I think, with that, we'll wrap up and close. And I'd like to thank each of our presenters today for your excellent presentations and for the excellent discussion-- Brad, Marinka, and Manolis. I'd also like to thank our video and communications team for running this webinar, and you, our viewers, for your attention and your participation. Please feel free to reach out to us. Thank you, and be well and safe.
MANOLIS KELLIS: Thank you, Sheryl.